
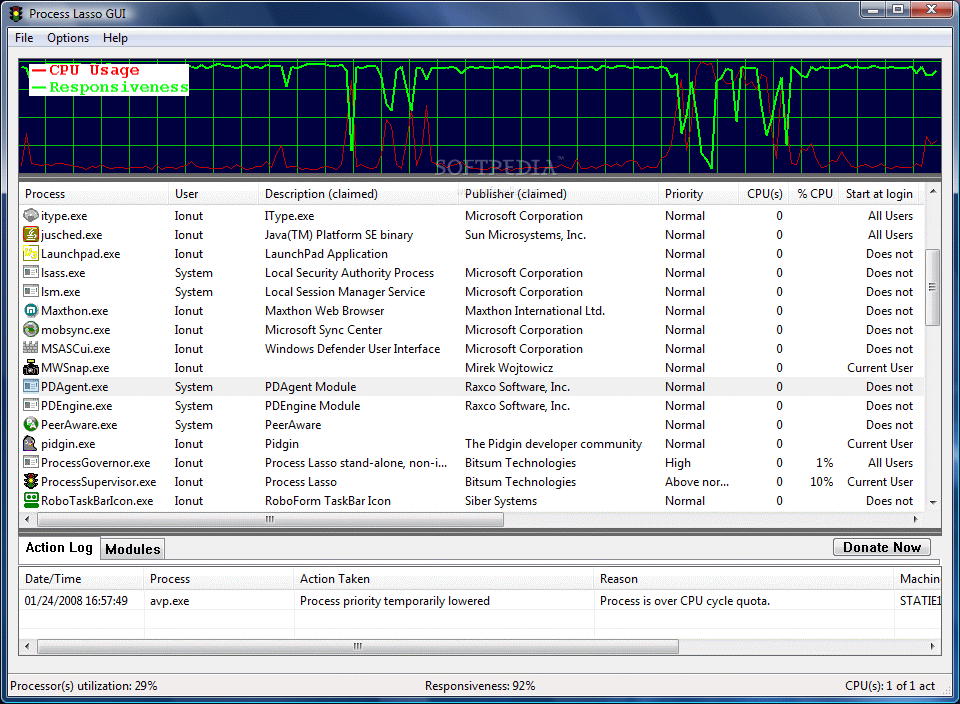
This is a very important concept in autoregression models and is also used in determining the order p of the autoregression model.

Remember, while learning about the AR (1) model, you had come across a term - autocorrelation.
AR PROCESSES LASSO PROCEDURE HOW TO
You must be wondering, how to determine the order p of the autoregression model? Later you will learn how to estimate these parameters using the ` statsmodel` library in Python. Determining these coefficients mathematically is little complex and is beyond the scope of this article. Some commonly used techniques are the Ordinary Least Square technique and the Yule-Walker equation. There are a number of ways available to compute the parameters 𝚽 p's. Here y t is assumed to have a correlation with its past p values and is predicted as a linear combination of past p values. This is the autoregression model of order p. , p) are the parameters of the model and c is the constant. Let’s see the form of the model when two lag values are used in the model. You can use more than one past term to predict the value at time t. In the above example, you saw how the value of y t is estimated using one past value (lag term), y t-1. Let us have a look at other orders of the autoregression model. You will be learning more about this later. Like the linear regression model, the autoregression model assumes that there is a linear relationship between y t and y t-1. The term autoregression means regression of a variable against its own past values. This is the autoregression model of order 1. Where 𝚽 1is the parameter of the model and c is the constant. You can estimate the relationship between the value at any point t, y t, and the value at any point (t-1), y t-1using the regression model as below. Let the observation at any point in time, t, be denoted as y t.
AR PROCESSES LASSO PROCEDURE SERIES
Instead of using a second time series (the stock price of BAC), the current value of the stock price of JPM will be estimated based on the past stock price of JPM. Now, let’s suppose you only have one series of data, say, the stock price of JPM. Where m is the slope of the equation and c is the constant.
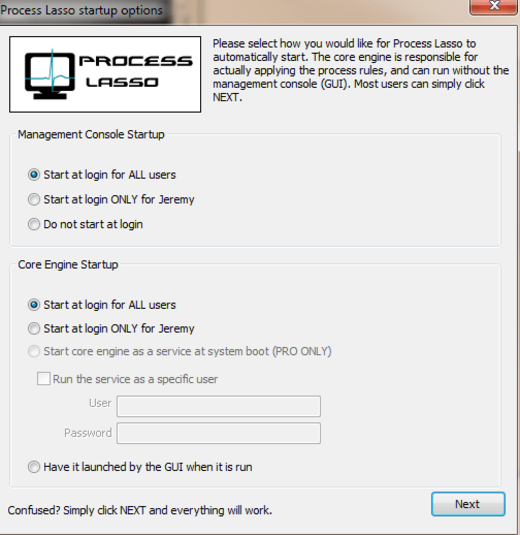
